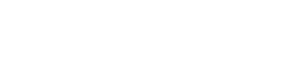
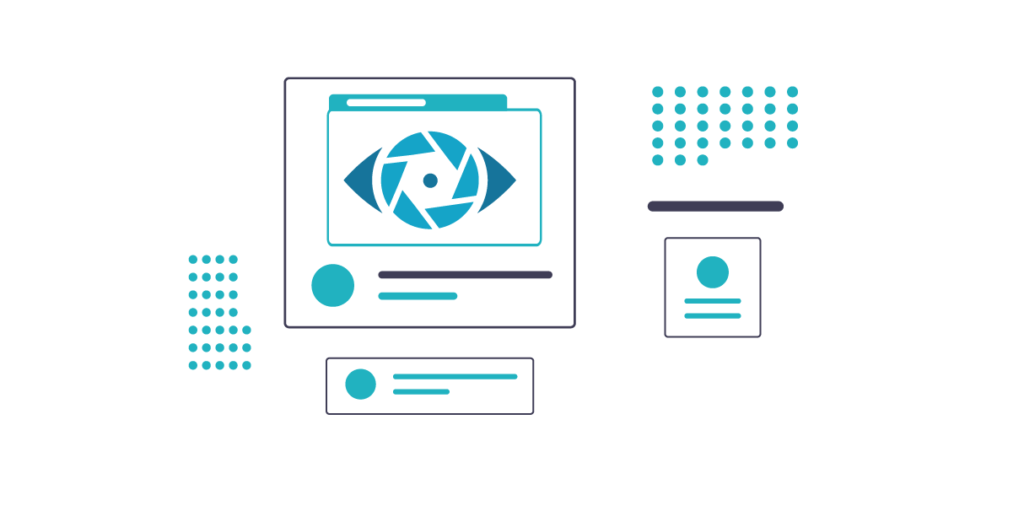
Deep learning machine vision software
Deep Capture is the most powerful deep learning machine vision software on the industrial image analysis market. It allows objects detection, analysis and classification. It is used on complex inspection issues to which traditional machine vision systems cannot respond (great diversity of references, objects with high light reflection). The software works in supervised learning (deep learning), from labeled images on the areas sought. Working on the best GPUs on the market, we are able to detect and process images in real time at framerates above 60 fps per camera.
Deep learning allows, from a dataset of images to be detected, to generalize the detection of elements not learned. In other words, our machine visionsystem Deep Capture is capable of detecting objects with a great variability of shapes, textures, dimensions … From a “hypermodel” created by our teams, the software allows fault detection on a wide variety of references without changing particular formats (no change in camera or lighting position) and freeing you from variations in exterior lighting.
Deep Capture does not require any special skills in machine vision or in deep learning to be used and has very few settings parameters. 2 automatic calibration phases upstream of the start of production will make it possible to increment the image model of objects without defects and then to define the camera thresholds per pass if necessary.
- Detection, classification and sorting of complex defects
- Moving objects tracking
- Complex character strings and OCR replay
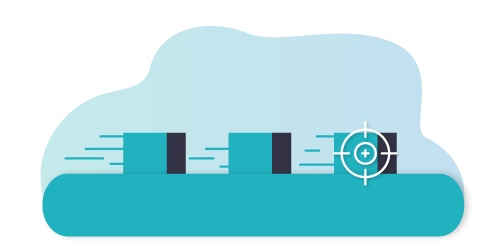
Operation
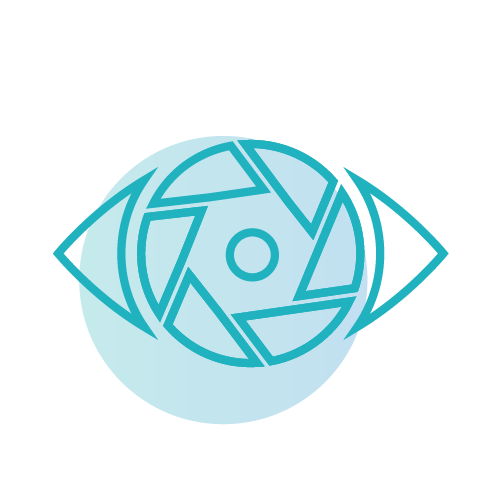
Simple and intuitive photo taking and labeling with DEEP VISION
Our machine vision software works in supervised learning. The system requires characterizing and annotating the elements sought in the images. This preliminary step is carried out on our Deep vision software which allows in a simple and intuitive way to create “bounding boxes” around the objects in order to accelerate and optimize the process, the labeling does not require to be very precise.
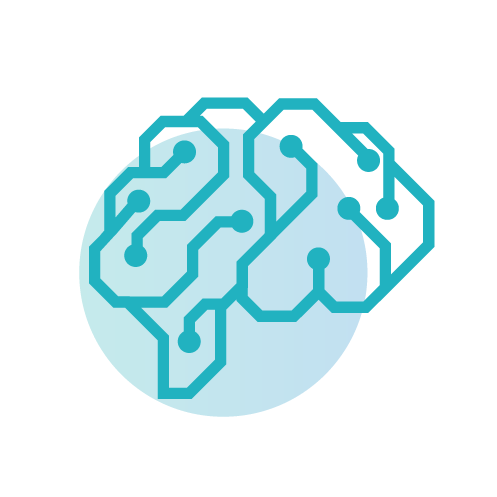
Model creation and training on the most efficient neural networks and frameworks on the market
Our models are trained on architectures proven on standard computer vision datasets. The model is compatible and trainable on the Google artificial intelligence platform: Tensorflow.

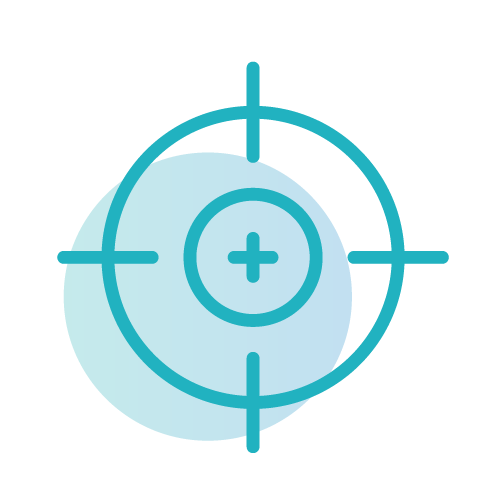
Automatic calibration
The tool allows thresholding of detected images. This threshold corresponds to the system’s “confidence level” on the given image on a scale of 0 to 100 (100 corresponding to the maximum confidence level). Our deep learning machine vision software enables the passage of N objects qualified as negative (not presenting any defects to be detected) to automatically calibrate the threshold per camera.
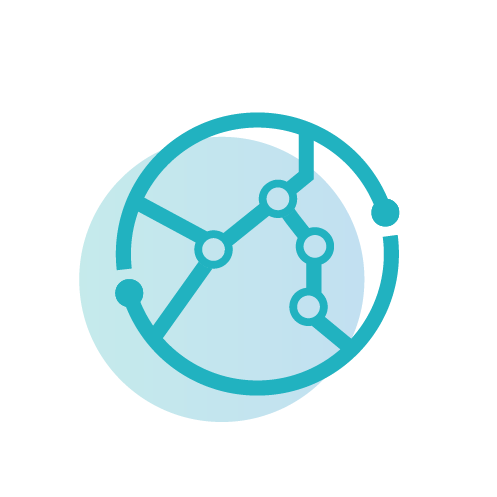
Strengthening the online model
A Deep Capture software feature allows the end user to improve the existing model and system performance, by automatically adding negative and positive product photos.
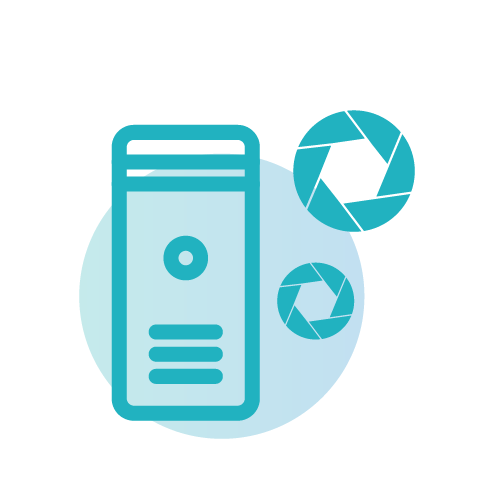
Ease of multicaming
Our deep learning machine vision software can operate up to 8 cameras on the same PC. Adding a new camera is easy and intuitive.
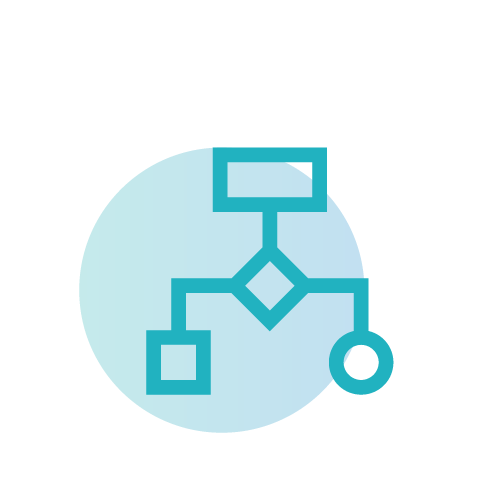
Decision support algorithm (svm, filters on the number of frames)
We have put in place a set of tools made possible by the fact that we capture and analyze images at high frequencies. The tracker is the simplest example. It only validates a detection when it detects the same object on at least n consecutive images. We have also developed a decision support tool. This tool is all the more efficient as producing a deterministic decision algorithm becomes complex and arduous as the number of objects that one wishes to detect increases. Our decision support algorithm uses the output of our deep learning model to produce its decision. If the detection model changes, it suffices to re-train this decision algorithm from the data already available to obtain an algorithm that fits this new model.
We also allow the user to modify in real time, and after the model has been trained, certain parameters (accepted threshold of confidence in the detection, number of consecutive images to consider a defect, objects superposition, image size considered by the neural network, camera settings, etc.). Our deep learning machine vision software is also capable of managing several detection models at the same time, which therefore makes it possible to adapt to unexpected situations (major products change on a chain, for example).